How to Detect Hate Speech with Machine Learning?
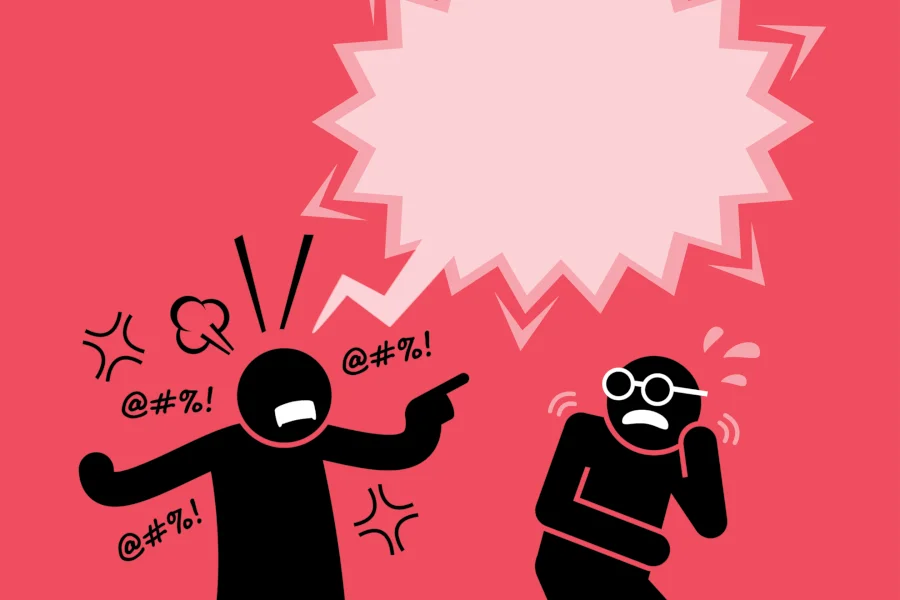
We encounter hate speech in every aspect of life, unfortunately. It is even more challenging to deal with its destructive effects in the digital world. People may act more aggressively on social media because they can be anonymous, their messages can reach a massive exposure, and of many other reasons. When we include the messages posted by bots and fake accounts, hate speech becomes too common to be detected and moderated manually.
In an environment where even the biggest media outlets in the world are sometimes forced to disable comments on sensitive videos they publish on YouTube, it is almost impossible to manually fight hate speech for companies and other organizations with more limited resources. Therefore, it is inevitable to resort to methods that automatically detect hate speech.
With the high capacity of computer processors and the power of artificial intelligence built on smart algorithms, machine learning technology comes into play at this point and offers solutions to those who want to fight hate speech.
Before we talk about how machine learning is used to detect hate speech, let us recall what hate speech is and why it is crucial to fight it.
What is Hate Speech?
According to the Cambridge Dictionary, hate speech is "public speech that expresses hate or encourages violence towards a person or group based on something such as race, religion, sex, or sexual orientation." In addition to this definition, the Council of Europe underlines that hate speech is more destructive when it spreads through the media and emphasizes that hate speech is a severe threat to democracy, multiculturalism, and pluralism.
Detecting Hate Speech With AI
To understand why fighting hate speech is essential for humanity in general, it is enough to remember the Council of Europe's emphasis on democracy, pluralism, and coexistence. For this reason, brands, especially media organizations, need to make sure that they do not mediate the spread of hate speech, even unintentionally.
This is why social media companies like Facebook, Twitter, and Instagram invest so heavily in solutions for detecting hate speech, preventing its circulation, and removing the related content, even if these platforms are not the original source. For instance, Facebook, which has 1.7 billion active users, has announced that it removed 9.6 million pieces of content it deemed hate speech in the first quarter of 2020, up from 5.7 million in the fourth quarter of 2019. According to the statement, 88.8% of these contents were detected and removed automatically by the software used by Facebook, before users reported them.
Likewise, while running a campaign, a brand must make efforts to ensure that they don't use hate speech -even unintentionally- or that the language they use does not provoke hatred.
The detection of hate speech is also vital for organizations that conduct studies on this issue, as it is already the subject of the research. For instance, if an NGO wants to investigate how a particular minority is perceived in the U.S. Therefore, being able to detect hate speech will be essential for this NGO. Political parties also benefit from this type of analysis to understand whether their discourses cause hatred in the society, especially when the elections are approaching and the debates are more intense.
What Are the Difficulties in Detecting Hate Speech With AI?
Even though detecting hate speech is a critical issue, it is not easy to do it efficiently and effectively.
First, the volume of the data that needs to be collected to obtain reliable results from the research makes it almost impossible to process this data manually. Classifying tens of thousands of lines in the database one by one as "hate speech" or "not hate speech" requires a tremendous amount of time and workforce. Going with smaller data to avoid this problem, on the other hand, cripples the reliability of the research.
Second, trying to make sense of the data with keyword-based searches does not give correct results due to the language's structure and forms of expression, such as irony. For example, a search with the word "gypsy" does not mean that all the results are hate speech. The phrases that criticize those who use this word as hate speech or that have nothing to do with the subject (such as comments about the movies “Time of the Gypsies”) cannot be classified correctly with this method.
How Machine Learning Can Help with Hate Speech Analysis?
Thanks to the high capacity of computer processors and the power of artificial intelligence built on intelligent algorithms, machine learning is used to eliminate the problems mentioned above regarding the detection of hate speech. If you need more information on machine learning and how it is used in qualitative research, please feel free to check those articles.
To use machine learning for detecting hate speech, the system must first be trained for how to recognize such discourses. For this, the data obtained from a study on the subject or collected from digital platforms are uploaded to the system. Then, the user examines the data line by line to label whether each content is hate speech. The more labeling is made at this stage, the higher the system's accuracy rate will be. Some products, such as Kimola Cognitive, also provide ready-to-use models, allowing the user to skip this labeling step.
After the training step, when the system encounters new data, it can detect whether it is hate speech by using what it learned during the labeling process for the previous data. The high processor power of the system makes it possible to finish the classification very quickly. Moreover, the advantages of smart algorithms come into play at this point.
As with the Hate Speech Detection Model included in the Cognitive product, the system does not focus only on keywords when classifying the data. It concludes by considering many variables such as which words are used together, where they are positioned in the sentence, and which punctuation marks are used. So, if the words "Gypsies" and "Time of the” are used together, the system can now make a classification for this because it has learned that this is a movie title, but not a hate speech.
How to use Cognitive's Hate Speech Detection Model?
Cognitive's Hate Speech Detection Model is a ready-to-use model prepared by Kimola. Prepared dataset for the Hate Speech Detection Model was trained with a freely available dataset consists of 2,545 social media contents labeled as "hate speech" and "not hate speech." Before the last version of this dataset prepared for the model was reached, the training dataset was tested with four different data consisting of random social media contents. Each wrongly labeled row was exemplified until correct labeling was achieved, and the training set was developed until it worked with a very high accuracy rate.
Step 1: Data Collection
To conduct reliable research on hate speech, a large volume of data is needed. For this, you can try Kimola's Analytics product, which profiles thousands of people anonymously in real-time through their social media activity and enables you to discover trends for different target groups you have created.
Step 2: Uploading Data by Choosing Hate Speech Detection Model
Next comes the uploading of your database provided by Kimola Analytics or from other sources. You can easily upload your data to Kimola Cognitive, which does not require any technical knowledge to use, is entirely web-based, and has an interface that allows the data to be uploaded by just dragging and dropping.
When you upload your database to Cognitive by selecting the Hate Speech Detection Model, the system creates a new column in your Excel file called "Hate Speech," analyzes the contents of each row, and classifies them as "Positive" or "Negative."
Step 3: Reaching Insights Based on the Results
After downloading the processed data to your computer, the human factor is back in the play. By examining the data labeled as hate speech, it is possible to see which words are frequently used as hate speech and to what individuals or groups they are directed at.
Moreover, if you have other categories in your database, using them together with the hate speech category allows you to gain priceless insights. For example, you can now see in which demographics hate speech is more common, which media platforms are the sources, and what type of motivation lies behind it. You can now decide what you’d like to do with these insights.
Let's say an NGO has conducted a study on refugees by using categories of demographics, age, and some other. They might conclude, "Hate speech against refugees has been spreading in smaller cities, mostly between men aged 18-24. This audience chooses Facebook as their primary news source and interacts more with the video content.” This valuable insight would allow the NGO to collaborate with the administration in those cities, create video content to soothe the hatred, and circulate it through Facebook.
Try Cognitive's Hate Speech Detection Model for Free
Having built on the machine learning technology, Kimola Cognitive allows you to classify your high volume data quickly and with high accuracy. Cognitive's Hate Speech Detection Model makes it possible to easily determine whether the data you obtained in your research involves hate speech.